Leveraging machine learning and predictive analytics for early detection of plant stress for the benefit of sustainability in farming
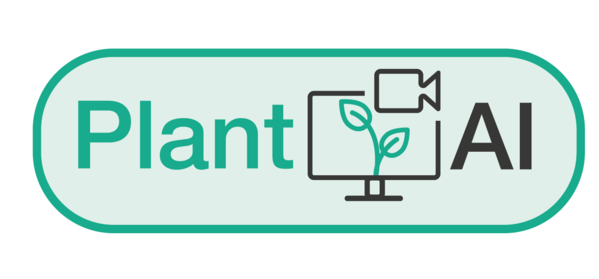
Opportunities through novel sensors
Modern sensor and imaging technologies provide promising means for measuring biological plant traits including abiotic and biotic stresses. Expectations are high that using these novel technologies in plant sciences will contribute to food security through novel breeding opportunities. Additionally, more precise monitoring will contribute to better assessment of ecosystem services valuation. However, the massive amounts of captured data resulting from modern sensors and their multimodal nature require advanced algorithms that are capable of identifying and retrieving patterns that relate to plant traits indicating, for example, biotic and abiotic plant stress. Currently progress is, however, impeded by a lack of suitable plant physiological reference datasets that allow for development of robust feature detection methods and reliable in-vivo plant diagnostics models. This project shall close this gap.
An interdisciplinary effort
In the project, a multi-disciplinary team of researchers has joined forces to foster research in the automatic identification of plant traits and the early detection and quantification of plant stress. We combine expertise from biology with that from computer science (i.e. machine learning, computer vision and statistics) for the development of novel analysis methods for plant monitoring data. By improving plant monitoring and early stress detection, we aim at contributing towards a more resource efficient future agriculture by e.g. more sustainable irrigation strategies.
Precise plant monitoring system
In the project, we will develop a precise closed chamber plant monitoring system that allows us to conduct systematic, precise and repeatable plant experiments and simulations. Massive amounts of data will be generated by continuously capturing hyperspectral image data as well as numerous parameters from the plant (e.g. leaf water potential and photosynthesis traits) and its environment (e.g. temperature, humidity, and leaf thickness). From this data a first comprehensive multimodal reference dataset for in-vivo plant diagnostics shall be constructed to foster research in automated plant trait identification. This dataset will include hyperspectral time series, as well as continuous measurements of plant-specific and environmental parameters.
Machine learning for recognition of plant traits and predictive modeling
To analyze the captured data and the relations within, we will develop novel data mining and machine learning techniques for the identification of hyperspectral plant patterns that relate to changes in environmental parameters. Furthermore, hidden relations between hyperspectral signatures and environmental influences shall be discovered to identify reliable parameters for the early prediction of plant stress originating from environmental conditions like water deficit.
The application of the novel approaches is demonstrated for the prediction of grapevine stress response using multimodal models based on spectral features combined with ambient parameters. It is expected that the novel analysis techniques will improve diagnostics of stress influence on the plant compared to simple vegetation indices and soil moisture-based thresholds commonly used in irrigation scheduling.
Benefit of the project
The project will provide a novel solution for generating high-quality physiological reference datasets for advanced hyperspectral analytics and enhance the detectability of high-level plant features. The obtained datasets, feature detection and pattern mining methods as well as the developed prediction models will be compiled into a publicly available library to foster comparability and reproducibility and to support different stakeholders involved in smart farming to optimize exploitation of hyperspectral sensor data.
You want to know more? Feel free to ask!
Media Computing Research Group
Institute of Creative\Media/Technologies
Department of Media and Digital Technologies
- University of Natural Resources and Life Sciences, Vienna, Department of Crop Sciences (DNW) Division of Agronomy (Lead)