Auditory Augmented Reality in Production (AARiP)
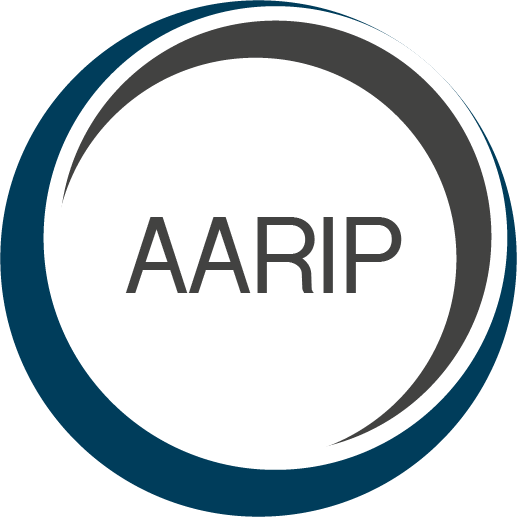
Duration: 01/01/2019 – 31/10/2019
The use case AARiP lays the foundation for the development of “intelligent” noise protection headphones within the framework of Cyber Physical Production Systems. The application is primarily intended for production environments with noise emissions above 85dB SPL where hearing protection is required. This means that specialist workers are not burdened with having to wear additional equipment but receive added value through the technological upgrade of their customary hearing protection. Even in production settings with low noise exposure, workers often wear headphones for listening to music. This constitutes another possible application area.
This is how the application works: Noises emitted by machines during production are captured via airborne sound microphones and structure-borne sound microphones and evaluated with the help of machine learning algorithms. The probability that a process works faultlessly can currently be ascertained with an accuracy of more than 93% in this context. This degree of error probability is used to control real-time sound manipulations (such as, e.g., pace fluctuations increasing in proportion to the probability of errors), which has a direct impact on the music that people listen to while working.
Another purpose in addition to the detection of errors is the acoustic representation of machine processes themselves, i.e., processes that function optimally are also represented in terms of sound (through playing music in an unmanipulated manner). In this case, AARiP refers to the occasionally underestimated “working knowledge” (also referred to as implicit or tacit knowledge), in other words, the experience that workers acquire after years of working with a machine.
Here is an everyday example to illustrate this: While driving, car drivers also pay attention to the noises emitted by the car (although they are not aware of this most of the time). Both the lack of an expected sound and the occurrence of an unusual sound would alarm them to the fact that, e.g., something may be wrong with the engine. We need “normal” sounds to confirm that everything is working the way it should. The use case AARiP takes advantage of this perceptual phenomenon. One challenge in this context was the development of a sound scenario which is both informative and acceptable to specialist workers over longer periods of time – and which, ideally, has a motivating effect.
Another two acoustic levels of information are planned for the future:
- Acoustically processed machine- and production-related data and key figures that are relevant for production (inventory, range, set-up, etc.)
- Wireless verbal communication with staff members
Conclusion:
- The probability of errors in a production process can be assessed in (quasi-)real time with an accuracy of more than 93% using machine learning algorithms based on sound emissions.
- The degree of error probability is applied directly to the sound properties of music transmission via headphones and can be clearly perceived by the users.
- Thanks to the continuous representation of information, workers can identify deteriorations of work processes early on and can take measures at a suitable time. This helps to avoid alarm messages that cause stress.
- Specialist workers can select their music as they like, i.e., our application serves to increase motivation and has additional information value.
- Further fields of application include use in control rooms and for training purposes.
Use case lead
- Academic Director GRACE - Gamified Reality Applications for Real-World Challenges and Experiences* (MA)
- Academic Director E³UDRES²
- Lecturer
- International Coordinator
- Department of Media and Digital Technologies
Team
-
Head of
Digital Technologies Research Group
Institute of Creative\Media/Technologies -
Researcher
Digital Technologies Research Group
Institute of Creative\Media/Technologies - Department of Media and Digital Technologies
-
Research Assistant
Digital Technologies Research Group
Institute of Creative\Media/Technologies - Department of Media and Digital Technologies