Multimodal information extraction and machine learning techniques for the extraction of real estate related attributes and parameters from heterogeneous input data
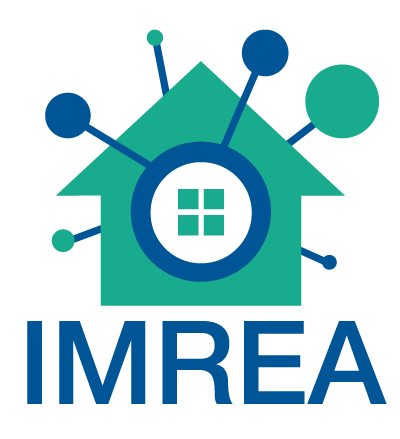
Background
Current real estate appraisal techniques require a great deal of manual data preparation and data processing and sometimes even on-site inspection. All this makes the process time-consuming and expensive. Input data for an appraisal often come from a rather small set of partly redundant data sources, which limits information content that can be extracted and as a consequence impedes valuation performance. Even for automated valuation models (AVMs) already available, input data has to be entered manually, which not only constitutes the largest cost in the process of valuation but also bears the risk of fraud (e.g. when data are entered incorrectly on purpose to create higher market values, leading to higher loans).
A largely automated approach that considers many different types of property-related data in a more complementary way is not available. Complementary in this context means that information is extracted automatically from and across different data sources (modalities). Such an approach would increase information content for each property significantly and would enable automated appraisals with higher accuracy. It is expected that in practice 50% of all real estate valuations could be replaced by automatically generated appraisals.
Project content
The IMREA (Intelligent Multimodal Real Estate Analysis ) project aims to develop multimodal information extraction methods that can deal with the complementary nature of real estate data. The methods will operate fully automatically, incorporate numerous complementary data sources (images, text, semi-structured data) and use multimodal machine learning models able to robustly predict a rich set of real estate related attributes (e.g. building size and building type). It will enable the extraction of metadata complementary to already available data and enhance data availability. This will improve reliability and forecasting quality of automated valuation models, thereby significantly reducing duration and appraisal costs in credit rating. The approach also enables continuous monitoring of the value of an object, making automatic early warning systems for large-scale real estate portfolios possible.
Project goal
Existing methods often operate on one modality only (i.e. mostly structured data) or model several modalities separately. The main goal of this project is the development of multimodal information extraction and machine-learning techniques for the robust extraction of real estate related attributes and parameters from heterogeneous input data. Such data will include structured text, unstructured text and images and build the foundation to generate more precise and up-to-date automatic real estate valuation models. Beyond this, we will improve methods dealing with missing data and missing modalities in machine learning, in particular we will focus on imbalanced multi-class learning problems.
You want to know more? Feel free to ask!
Media Computing Research Group
Institute of Creative\Media/Technologies
Department of Media and Digital Technologies
- University of Applied Sciences Kufstein (lead)
- University of Applied Sciences St. Pölten
- DataScience Service GmbH