Active Machine Learning for automatic identification of handwriting in 12th century manuscripts
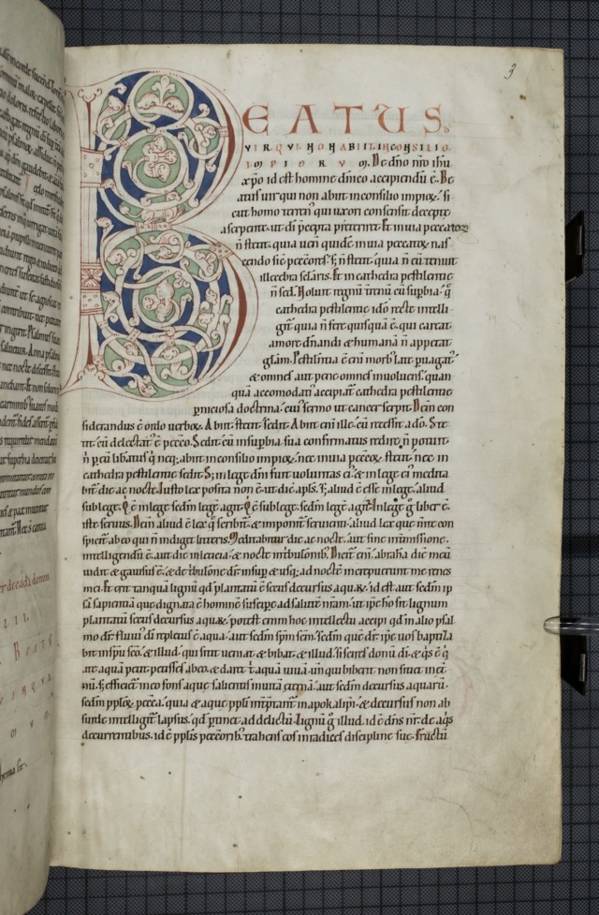
Monastic scriptoria in high mediaeval Austria
Lower Austria’s monasteries have extensive collections of medieval manuscripts. A broader knowledge about scribes enables a better understanding of monastic scriptoria in high mediaeval Austria. However, there is no information about the number of scribes employed and whether they moved around between monasteries. A way to determine these factors is by analysing writing styles to identify different scribes in a large number of manuscripts by inherent stylistic characteristics of their handwriting. This allows to deduct the whereabouts of the scribes and the organisation of the scriptoria.
Using Active Learning to identify different scribes
Usually medieval handwriting is analysed by individual experts which is a lengthy and time-consuming process. Furthermore, it carries the risk that results are biased as these are subjective impressions of individual experts. First approaches to support the identification of mediaeval writing hands by machine learning exist. However, these are not suitable for large corpora, the main challenge being the lack of extensive baseline (ground truth).
Goal
This interdisciplinary project involving historians and computer scientists aims at developing time efficient identification of scribes for large corpora. It deploys an active machine learning approach that specifically involves human experts to support the machine learning.
Method
Digitised manuscripts of the Klosterneuburg Monastery’s library serves as database. From this corpus, a dataset (Ground Truth) of about 3150 text pages annotated with scribe assignments is created. Based on this Ground Truth a classification model for the identification of scribes is developed and trained. Classical descriptors are supplemented or replaced by automatically learned descriptors (Deep Learning).
In addition, there is a corpus of about 40,000 digital manuscript pages with as yet unknown writer identification. This data source is transformed into data sets and subjected to an Active Learning approach. The classifier trained on Ground Truth can now make a preliminary writer identification and present any possible hits to palaeographically trained experts through an interface. Based on the expert evaluation, the model is improved iteratively.
Results
This project helps not only to work on a significant desideratum of historical research in an interactive way, but also establishes new possibilities and tools for analysis that allow a deeper knowledge of all other medieval scriptoria in today's Lower Austria. Based on the study of the Klosterneuburg scriptorium in the last third of the 12th century, larger unresolved questions about the organisation of scriptoria in the high medieval (Lower) Austrian monasteries can be addressed with further evidence and interpretations.
Publications
- Stift Klosterneuburg
- TU Wien