Automatic gait pattern analysis for robust classification of functional deficits. Intelligait aims at developing automated methods to analyze and classify gait patterns.
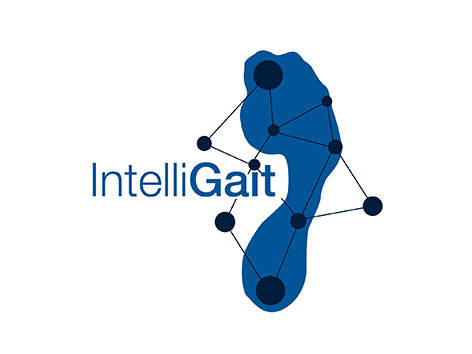
Background
A rising number of people in our community, especially elderly people, suffer from gait disorders and gait deficits. Most clinics and hospitals have to treat a large number of patients within a short amount of time and therefore typically rely on force plates and cost-effective two-dimensional gait analysis tools to determine ground reaction forces (GRF) and gait kinematics during locomotion to quantify the patients’ gait disorders and to evaluate the patients’ progress during physical therapy. From the shapes of the GRF signals and from visual observation the expert tries to diagnose whether a functional deficit exists and which type of deficit it could be. The manual analysis of gait patterns by visual observation and by visual inspection of GRF signals however, leads to subjective and non-repeatable assessments. Furthermore, it has been shown that several parameters (e.g. walking speed, age, gender) influence the shape of gait patterns significantly and further impede the manual (visual) assessment and comparability of different patterns.
Thus, more objective means to support the therapist in the assessment of gait patterns are required. Automatic analysis methods enable novel ways to obtain objective and repeatable assessments and thus represent a valuable supporting tool for the expert in making diagnoses. Recently, there has been an increase in research on the automatic analysis and classification of human gait. From related work, however, we observe that the performed analyses and evaluations are often applied on rather small (and artificial) datasets. Thus, the general applicability of existing approaches in a real-world scenario is limited.
Research Goals
The Intelligait project is provided with a large-scale database of real-world gait patterns gathered during clinical praxis. This dataset enables us to develop novel methods for automated gait analysis that take the real-world requirements more adequately into account. The contributions of the proposed project are:
- The development of robust normal behavior models that are invariant to different categories such as walking speed, age, gender, and physical condition of the patient to enable the distinction of normal from abnormal gait;
- The introduction of novel features for gait data that derive directly from the data. The novel features allow for the classification of a wide range of functional deficits and should support the expert in knowledge discovery;
- Data-driven access to gait pattern databases enables immediate access to a much larger data base than currently available.
The vision beyond this project is to develop methods which are capable of effectively supporting medical specialists in making decisions, increasing patient throughput and in consequence reducing medical treatment costs.
Project Partners
The project is carried out in cooperation with the Board of Compensation for Austrian Workers’ (AUVA NÖ), which maintains an extensive data collection of clinical instrumented gait analysis. The University of Vienna consults regarding biomechanics. The St. Pölten UAS study programme Physiotherapy contributes clinical expertise, while the IC\M/T provides know-how regarding signal processing and machine learning.
Video
- University of Vienna, Departement of Sport Science, Biomechanics, Kinesiology and Computer Science in Sport
- the Austrian Workers' Compensation Board (AUVA)